
Ranking questions are used when you need to distinguish levels of preference in a list of options. This applies to questions where determining the priority, and not just the number of times an option is selected, is important for your analysis.
Many times, when we find that there isn’t a need to distill the results down to a level of preference, we advise sticking with a multi-select question instead.
We elaborate on the reasons why below.
Multi-select can give us ranked data by comparing the percentage fall out
Often people default to a ranking question because they want "ranked data", but end up conflating their needs for a question type with the desired data structure.
The thing is, multi-select questions can also give you a “natural ranking”. The higher the percentage selection, the more popular the option is, giving you the ability to derive a ranked order from it. Additionally, you get a sense of how much more popular an item is over another by comparing the percentage fallout. With ranking, we typically reduce the insights to just ranks without getting a sense of how much they differ from each other.
To illustrate this, let us take a look at how the data differs for the two question types.
Here is an example dataset from our dashboard. The below question type is a Multi-Select question type. Here respondents were allowed to choose multiple answers (up to 5). The results show how popular each option is and you get a natural rank order based on the percentage fallout. You would interpret the data as : Location is ranked highest with 84% of respondents stating that it is an important factor when when buying a home.
Image 1 : Data for Multi-select question type

The image below is a Ranking question type (with weighted ranks). Results are weighted by assigning a rating score to each rank. Options that are ranked higher across different ranking positions will show a higher overall weighted percentage. Many often used the weighted ranked data (image 2) to summarise the results. However, one needs to be cautious in terms of how you interpret the data. You may conclude that overall Price and Location were both ranked highest but it would be erroneous to say that 24% of respondents selected it as the top factor.
Image 2 : Data for weighted Ranking question type
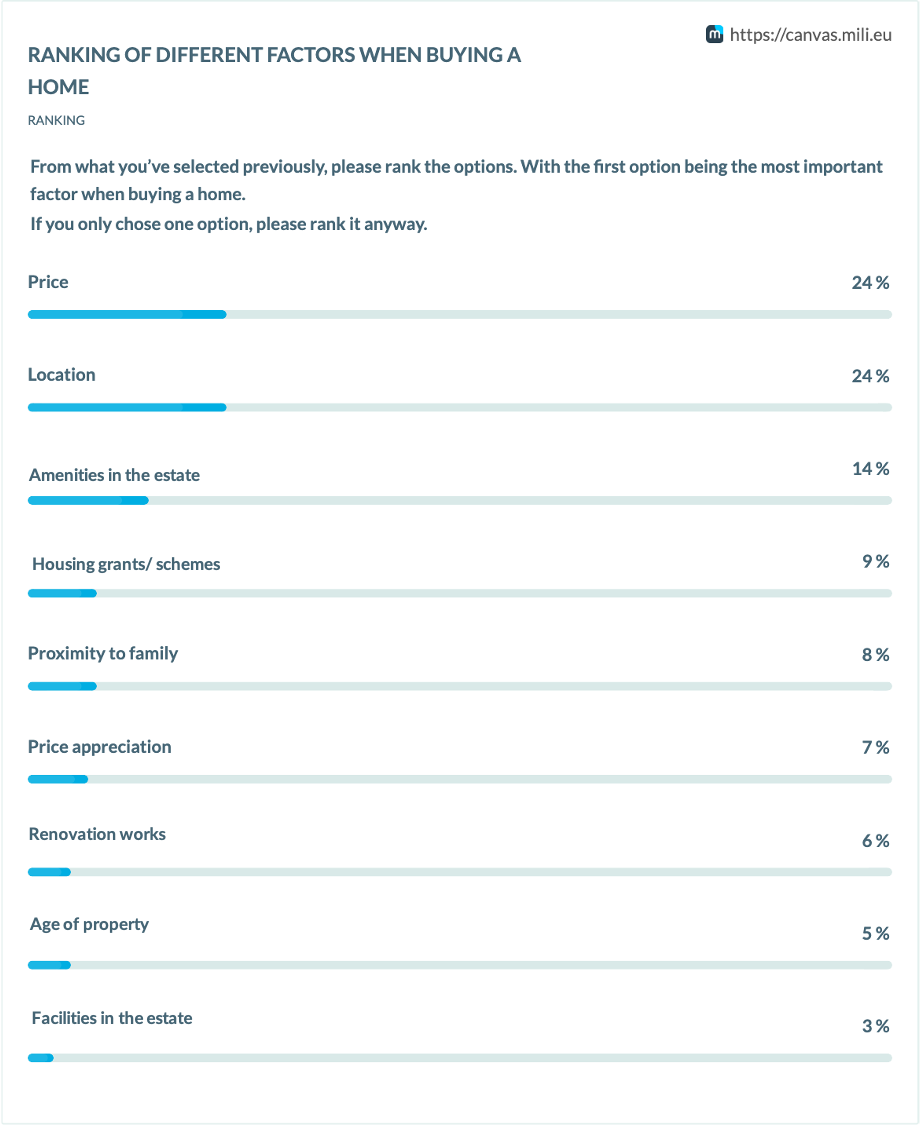
Multi-select reduces unnecessarily complicated data
Most importantly, results from multi-select question types are straightforward to unpack. On the other hand, ranking data introduces complexities in analysis that you wouldn’t get with a multi-select. For example, instead of just having a rank order of attributes/brands, you now have a ranking of those that were ranked 1st; a ranking of those that were ranked 2nd, and so on. Essentially, you get ranks within ranks (as can be seen in image 3 below).
Image 3 : Rankings within ranking question type result
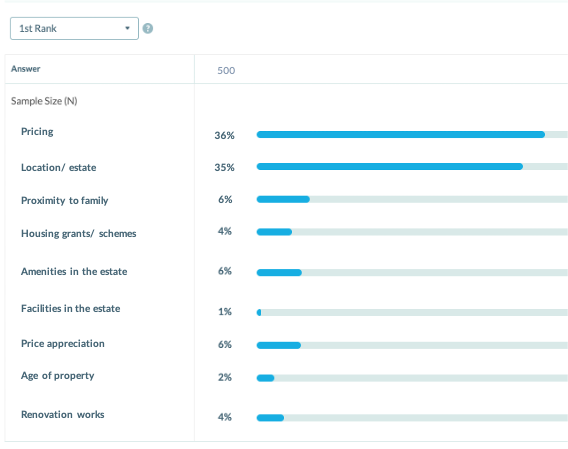
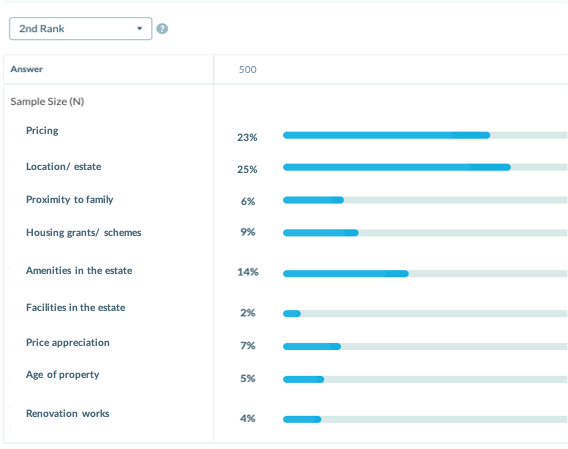
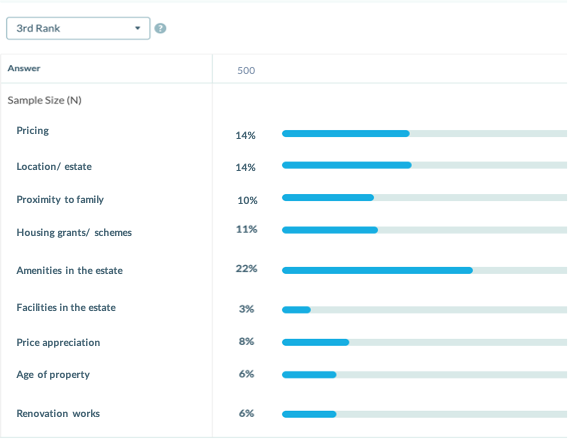
So before defaulting to choose a ranking question, ask yourself:
1. How do you intend to present the data? Is getting a top 3/ top 2/ top 1 read of the data enough for you?
2. Would identifying ranks within ranks be required in your analysis?
3. Are you okay with summarising the results using the weighted ranking approach?
How to best use a ranking question?
Now, if you do decide to use a ranking question, how best can we use it in a way that allows respondents to answer the question more decisively?
Here are some best research practises to get cleaner data.
1. The lesser the better
While it’s good to be exhaustive in your list of shortlisted options, we have to put ourselves in the shoes of a respondent. A general rule of thumb is to cap a ranking list to a maximum of 5 options. This reduces the cognitive load required to comprehend the question and options.
Respondents typically have stronger opinions about their top and bottom choices. So when the list gets too long, the options to be ranked in the middle may not receive the same attention. It runs the risk of being treated as an afterthought, as users are tempted to randomise the options which may compromise the quality of responses.
For instance, if you ask respondents to rank their favourite colour from a long list of say 10 options they are most likely to have a strong opinion on their favourite and least favourite colours. For the colours in the middle, they are likely to randomise the rankings as they might not have very strong preferences on their ranking order. Hence, it is best to cap it to 5 options where you can be more certain that they will answer with careful consideration.
When we reduce the need to scroll through a long list of options, it becomes less frustrating for a respondent to answer, and we can be more confident in the quality of the data.
2. Narrowing down an option list for relevance
Ranking questions are most effective when an initial list of options is narrowed down to a smaller list. This helps to keep the options list succinct and relevant to each respondent.
We’d suggest asking the question in 2 parts, especially if your list is long. The first part can be asked as a multi-select question with the full list of options and imposing a maximum number of options one can select (e.g., choose up to 5 options). For the second part, ranking can then be used in a follow-up question, showing only options that have been positively piped (i.e. selected options) from the previous question.
Ranking from a piped list prevents a respondent from ranking an option they wouldn’t even have considered in the first place. For instance, if you ask respondents to rank their favourite travel destinations, it doesn't make sense to have them order a list with some options that they may not have even heard about or traveled to. Narrowing down a list helps to prevent a respondent from being forced to have an opinion, and no one likes being forced!
Milieu Insight is a celebrated online survey tool and top market research agency, empowering businesses to succeed in a data-focused world.
Our brand health research guide highlights the significance of brand analysis in understanding consumer perceptions, navigating competitive landscapes, and formulating effective marketing strategies, showcasing innovations like diverse audience engagement and adaptable methodologies.